In 2016 Microsoft experimented in the ‘conversational understanding’ realm with a Twitter chatbot it called Tay. The aim was for Tay to draw on public data and scour Twitter to build on its library of knowledge… the more people chatted with Tay, the smarter it would get. Well, that was the plan.
Within a day of launching, Tay was spouting rather a large proportion of racist, misogynistic and nonsensical remarks among its 96,000 tweets. Some were a simple echo of the trolling messages that mischievous Twitter users had sent; others seemingly constructed from some of the nastier utterances from deep within Twitter’s databases.
“When AI gets wired on something that then scales, the impact can blow out quite quickly,” says Daniella Aburto Valle, founder of the Australian chapter of Humans for AI, a group of experts in data science who aim to bring diversity to AI development.
This example of good AI gone bad speaks volumes to the speed at which technology can pick up information and run with it, but is it always a good thing? And what part does the human element have to play in learning for the future?
It’s an exciting and hugely disruptive time for organisations and for societies globally as we face the ‘Fourth Industrial Revolution’: a nexus of technology where ubiquitous mobile communication, machine learning and AI, robotics, the Internet of Things and fast-advancing science herald an increasingly complex future.
Humankind has finally worked out how to outsource thinking. Now that we can teach machines how to learn, AI can help us fast-track all kinds of work and achieve goals previously impossible.
Service organisations are already seeing disruption as chatbots become skilful enough to serve customers – and this is just the edge of the wedge.
Capturing the magnitude of this opportunity sits in understanding nuance. Machines learn differently to humans – and understanding the intricate differences between how machines and humans synthesise new information is one key to finding new roles and the right application for each in tomorrow’s workplace.
Dr Amanda Allisey, Principal Consultant at Maximus, works with organisations at the cutting edge of introducing new technologies to the workplace, and believes that the advent of intelligent machines opens up opportunities for more interesting and diverse roles for human workers within organisations.
“The challenge for leaders is to find ways to create environments where humans thrive, and to help humans create ways of working that extend and leverage organisational performance,” says Allisey.
As an organisational behaviourist and lecturer at Deakin Business School, Allisey focused on leadership and resilience and says that any successful integration of technology in the workplace has to start with the humans, their mindset, behaviour and capabilities.
“In order to predict, influence and adapt human behaviour within the workplace, we need to have a deep understanding of how humans learn, grow and evolve,” she says.
With human experience as the central point, we can work out how technology can create differentiation in the market and create value to the work that occurs within the organisation.
“The relationship between humans and their environment will change to include a new intermediary: machines that can learn and can perform ‘thinking’ tasks for humans. The architecture of how this plays out sits in the hands of the human,” says Allisey.
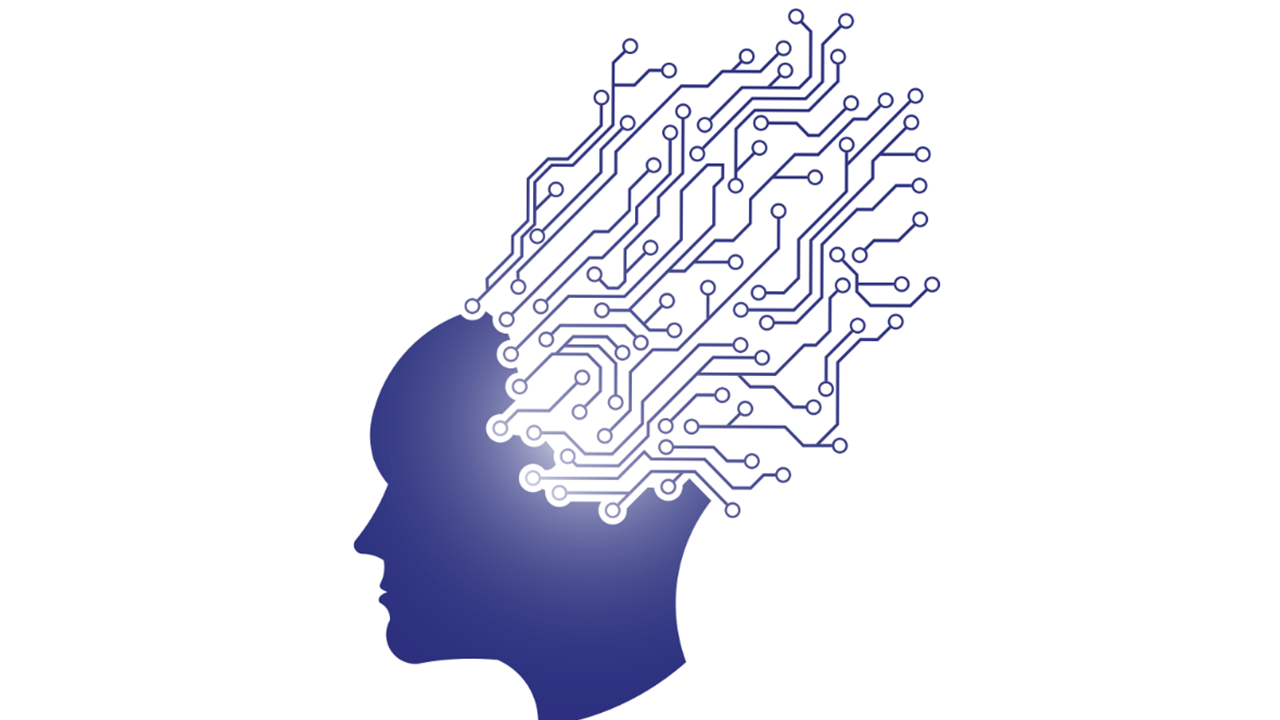
MACHINES WITH BRAINS: SIMPLY EXPLAINED
Those outside the computer science field often use the terms AI and machine learning interchangeably, but there are some distinctions between the two – and some experts also differentiate between several levels of AI.
Machine learning involves programming that uses neural networks – a set of algorithms designed to recognise patterns.
In machine learning, a human must program the information into the machine, select the data and run hours of training programs, then test the computer’s response and fix any problems spotted in the data.
Netflix and Amazon use machine learning to make suggestions for movies or products that you might like based on your previous choices, for example. But machine learning can only choose a solution based on data it has received – so outcomes are often literal, small-minded and annoyingly pedantic.
AI is one step beyond machine learning, taking data that has been learned, including past experiences, and then changing its own future behaviour. Examples include speech recognition, which improves as it becomes accustomed to your voice, and image classification – like the photo tagging in cloud-based programs such as Google Photos and Pinterest.
Various experts suggest three different ‘types’ of intelligence: artificial narrow intelligence, which can only do one thing; artificial general intelligence, which can do a broad range of things (as a human does); and artificial superintelligence, which – at this point – is a hypothetical final step in AI.
“We are giving leaders the exposure, insight and learning to work across this continuum. They must have a curious digital mindset and be able to understand and enable the application of these capabilities to their unique business requirements,” Allisey explains.
THE DIFFERENCES INFORM THE OPPORTUNITIES
We must move beyond the narrative that machine learning and AI will step in and take over tasks that humans perform. The narrative should recognise there is just so much that machines cannot do. It must focus not on loss but on leverage, opportunity and responsibility.
Humans can take intuitive leaps, connect seemingly disparate bodies of knowledge and draw meaning from them. We are programmed to ignore many distractions in our environment, allowing us to focus on the things that matter.
That makes humans less capable of painstakingly sifting through large datasets and applying consistent rules to each – we get bored and make mistakes. Humans are far better at responding to a new event, drawing on a vast range of experiential information and applying it.
Research shows that many human behaviours are instinctual. “Infants, for example, have innate moral judgment and can recognise right from wrong,” says Allisey. Humans also rapidly assimilate many disparate social cues to assess mood. “Most people can walk into a meeting and instinctively recognise whether people are hostile, welcoming or indifferent.” “Humans use context in order to learn,” Allisey continues. “A human can extrapolate based on prior experience. We make models in our brain that we use to interpret future situations.”
Computers learn in a far more linear way, using ‘brute force’ of sifting through vast amounts of data and information as fast as possible to solve a problem with the most likely probability. There have been various attempts to design an AI that learns in a more human way.
One example is Google’s DeepMind neural network which includes AlphaGo, an AI program designed to play the board game Go. It used a supervised learning protocol, where it analysed large numbers of games that humans played against each other.
In 2016, AlphaGo beat Lee Sedol, the human world champion Go player, in a five-game match, and AlphaGo has used its learning from Go matches to regularly beat itself in games of Go – and extrapolate these lessons to games of chess.
DeepMind is now using reinforcement learning, where it combines a policy network – which assesses probabilities – and a value network, which assesses its own performance.
Machines – at present – have far less to work with than humans, who are still far quicker to make snap character judgements and identify creative new solutions.
But because machines don’t filter out much of the extraneous ‘noise’ which humans ignore to make quick judgements, they are very good at assembling meaning from huge datasets. For example, in 2018, DeepMind’s AlphaFold program solved a huge challenge in science in helping to predict 25 of 43 protein structures based on amino acid sequences.
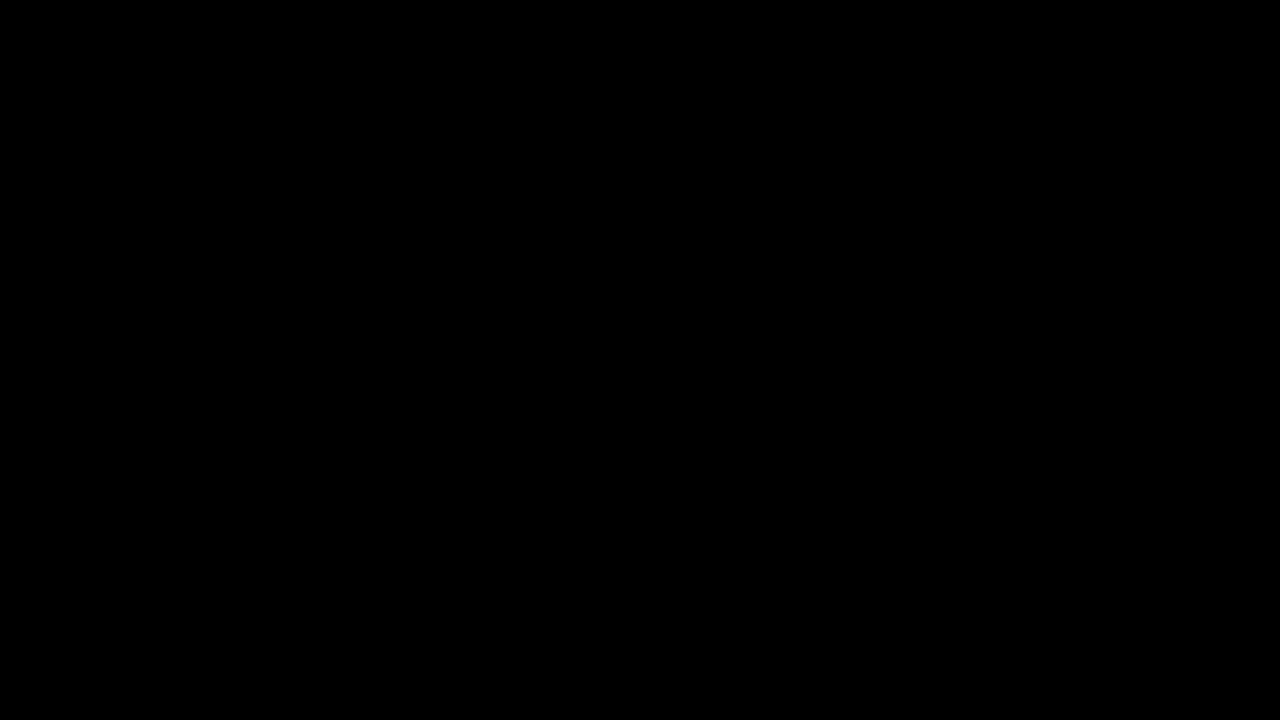
“A human can extrapolate based on prior experience. We make models in our brain that we use to interpret future situations.”
Dr Amanda Allisey, Principal Consultant at Maximus
AI FAIL IS ALL AROUND US
Author and AI research scientist Janelle Shane says AI is already everywhere – and one of the ways to work out how to use it best is to find out where and how AI performs at its worst.
In her new book, Shane writes that AI “… shapes your online experience, determining the ads you see and suggesting videos while detecting social media bots and malicious websites.”
The book, You Look Like a Thing and I Love You, released in November 2019, has emerged from her blog, AI Weirdness, which documents interesting experiments with AI.
“Companies use Al-powered resume scanners to decide which candidates to interview, and they use Al to decide who should be approved for a loan,” Shane writes.
However, she warns, AI is not flawless. “Ad delivery haunts our browsers with endless ads for boots we’ve already bought. Spam filters let the occasional obvious scam through or filter out a crucial email at the most inopportune time.”
One of the many examples of AI fails that Shane lists on her blog includes the time when she ran a photo of Darth Vader through an automated image recognition program. (“It declared that Darth Vader was a tree and proceeded to argue with me about it,” she writes.)
“Pranking an AI – giving it a task and watching it fail – is a great way to learn about it.”
KEEPING TABS ON DIVERSITY
Daniella Aburto Valle’s expertise in the merging of data science with large-scale renewable energy development saw her engaged in a major project with GE Digital, where complex software algorithms were used to optimise equipment life expectancy in wind farms.
Valle says that it’s early days in business, and most applications involving machines that learn operate in data-rich environments where machines don’t need to move beyond their programming.
“The mechanical, repetitive jobs are the low-hanging fruit when it comes to automation; inputting data, cycling information from one place to another – we are already seeing that, because the cost-benefits are really compelling,” she says.
She believes that as a society, we need to think both strategically and ethically about the jobs that will provide opportunities for the next step: AI where machines use previous interactions to improve future decisions.
The dilemma is that AI can reinforce and amplify undesirable biases, she says. “As you leverage AI, you’re wiring certain responses and creating algorithms that harden a specific point of view or a response that you have.”
This is because both AI and machine learning are based on huge volumes of existing data. Research shows that when AI builds algorithms for further learning based on real-world data, such as Google news, it will replicate and then amplify stereotypes – such as associating women with domestic chores and men with sports.
Another example is a chatbot, created using online responses. “If those responses are created taking in mind only one population or one perspective, then you’re hardening that bias,” says Valle.
These examples all show that without an instinctual moral sense, nor an ability to make social judgements from disparate information, machines are likely to need human intervention for the foreseeable future.
Humans for AI helps organisations develop AI that includes diverse views from the outset and which employs strategies to avoid shutting out large sectors of the population. For example: there’s extensive work on voice recognition so that it recognises people speaking English as a second language.
“Human-centred design is the key,” says Valle. “Putting your customers front and centre, and making sure you understand how they’re going to be experiencing your product – a chatbot, for example – and how you can make it more eloquent.”
Whatever the development, Valle believes there will always need to be a place where a human steps in. “Having humans in the loop allows you to have an intervention with that linear thinking of AI,” she says.
“The key difference between the way that machines learn and the way humans learn, is that humans form mental models, using innate learning, emotion, context and concepts as well as past experience,” says Allisey. It’s important to remember, adds Allisey, that humans created machines, based on our own fundamental understanding about how we learn.
This article was originally published in the 4th edition of M Magazine, an exclusive print magazine aimed at inspiring and driving change through Australia’s executives and heads of HR.
Related Insights
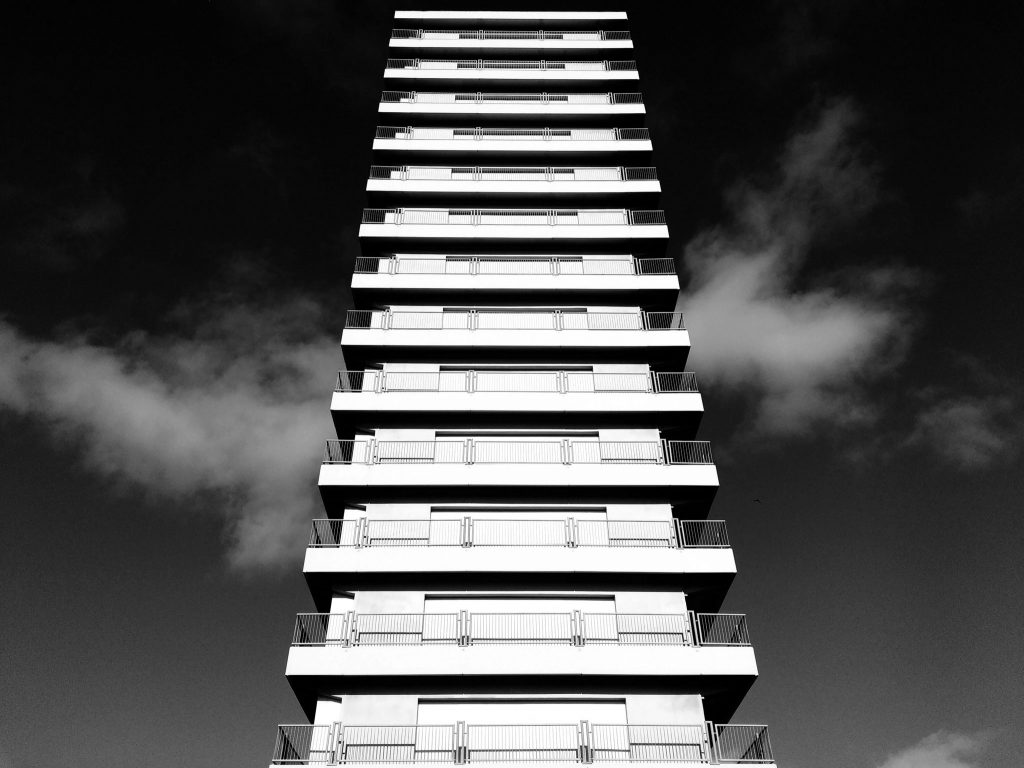
The Changing Landscape of Sales Leadership
On my recent holiday I read Dan Pink’s new book, To Sell is Human. The chapters argue the growing importance of sales skills in both traditional and non-traditional sales roles. Dan’s perspective runs against the viewpoints of many that believe the art of sales is in decline. In a world full of information that is so easily accessible through technology, many think digital and social marketing is replacing the role of the traditional “salesman”
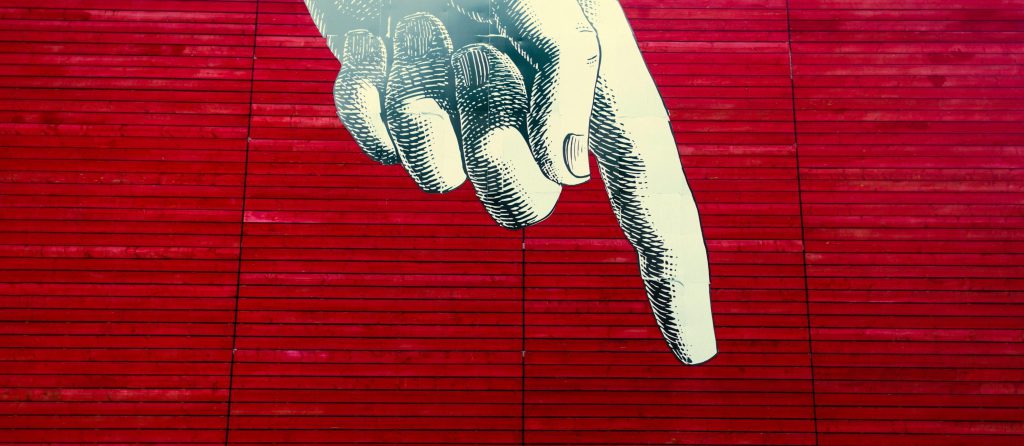
4 Essential Behaviours of Modern Leaders
Your management style is a reflection of your personal strengths, weaknesses, attitudes and the values that you have build up over the course of your life. Because of this, there are as many kinds of leadership as there are leaders. From the autocratic to the democratic, from the conceptual to the task-oriented, managers come in all shapes and sizes – with varying levels of effectiveness.
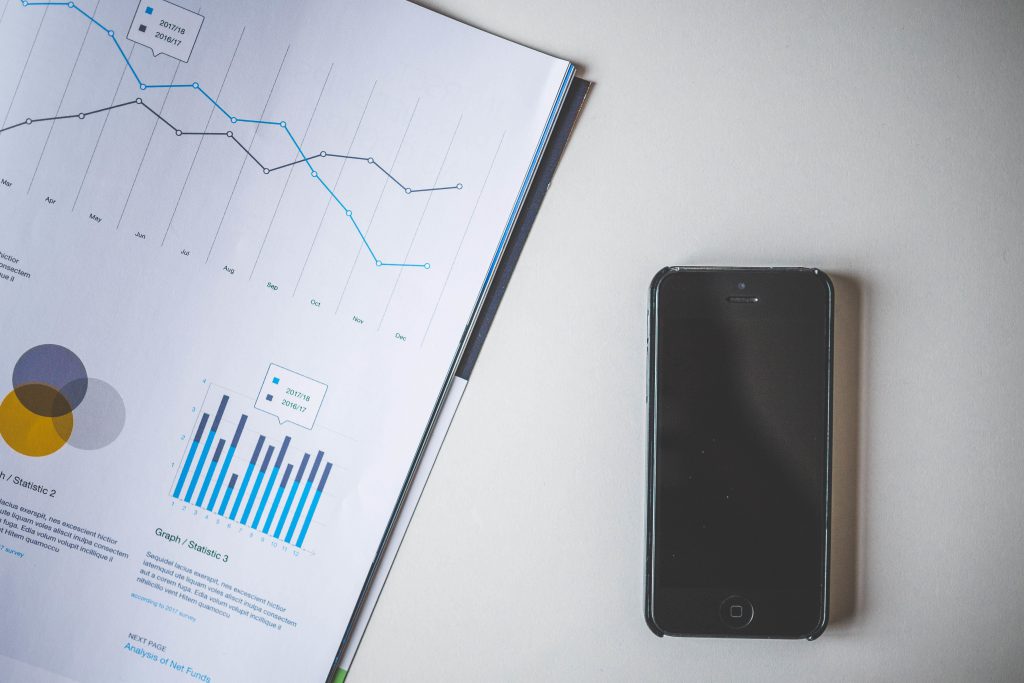
Performance Management Has Failed
Human resources has gotten caught up in a flurry of systems and processes. That overzealous desire for order and regulation belongs anywhere but in an organisation’s social hub. Excuse the psych jargon, but this is a prime example of Stratified Systems Theory. In other words, processes that are fundamentally human are getting policed with too much structure and complexity, making them disorienting and ineffective.